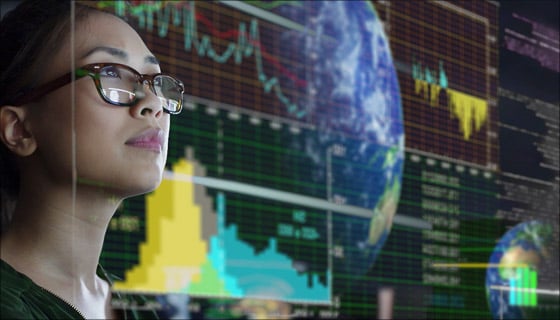
The Big Picture
Predictive analytics involves just that: prediction of the future. It is an inherently uncertain activity. While there are any number of metrics in analytics that can convince that a predictive model will indeed provide useful information, nothing proves the value to an organization as much as a verified success story. In addition, even good predictive models can begin to deteriorate over time as the data on which it is based gets older and older. A need exists to track this to know when to update a model.
The best way to verify the functioning of a good model and to know when it needs to be refreshed is to monitor that model’s business performance. (Note that we are not here discussing the technical performance – response times, etc.) In this installment, we consider the unique needs of monitoring operationalized predictive models.
Monitoring Requirements for Predictive Analytics
First and foremost, it is important for insurers to understand that the resources they will have to commit to any predictive model extend beyond the building and implementing phases. Any predictive model on which a business process depends must be monitored for effectiveness. Work done to monitor and refresh models must be accounted for and not seen as expenses to be shoehorned in.
Second, monitoring should be split into two pieces: distributions and performance.
Insurers’ books of business do change over time and verifying the similarity of the current production data against the historical data used to build the model is an important first step. Are the distributions in the data – age, limit choice, cause of loss, geographic region, etc. – similar in actual practice to the assumptions made when the analysis took place?
It is a separate question to ask if a collection of predictions proves to be accurate. A distributional shift to older policyholders does not necessarily mean that a model’s predictions on those policyholders are now incorrect.
Though a separate question, the consistency of the distributions of data is often a leading indicator to the model performance. Especially when a prediction involves insurance losses, it can be impossible to measure model performance immediately and having information about distributions is better than having nothing.
In addition, monitoring distributions can provide a safeguard against sudden changes in the data. Not only do insurers’ books of business gradually change over time, but choices about what data to collect can change as situations and strategies change. Consider an insurance regulator that decides to mandate a change to minimum limits and a predictive model which relies on limit as an input. This will show as a sudden change in the distribution of limits coming through production data. Monitoring of distributions allows an insurer to recognize the disconnect when it happens and take action to correct the discrepancy immediately.
Guidewire’s Approach to Monitoring
Guidewire’s MONITOR application is a distribution monitoring application directly integrated into the model deployment approach. The ability to monitor distributions in real-time comes automatically with the use of Guidewire’s deployment path, even for models built externally to the Guidewire platform.
Currently, the ease of extracting data from Guidewire’s data platform facilitates the monitoring of the performance of predictive models when the time is right. The BUILD application includes tools for applying existing models to newly imported data to see how well the model predictions track with observed results.
Perhaps more importantly though, Guidewire’s entire ecosystem provides the building blocks needed for real-time monitoring of model performance. This goal requires daily processing, data capture and storage, and predictive model details to be integrated in a way that policy or claims experience can be tracked over time after model predictions have been made.
Guidewire appreciates that monitoring predictive models needs to be real-time and is more complicated than it may appear. It is not an optional activity and should be integrated with the entire predictive analytics vision. Stay tuned for the last installment of this series on Guidewire’s vision for analytics. Also, don’t miss the previous installment discussing the implementation needs of predictive analytics.